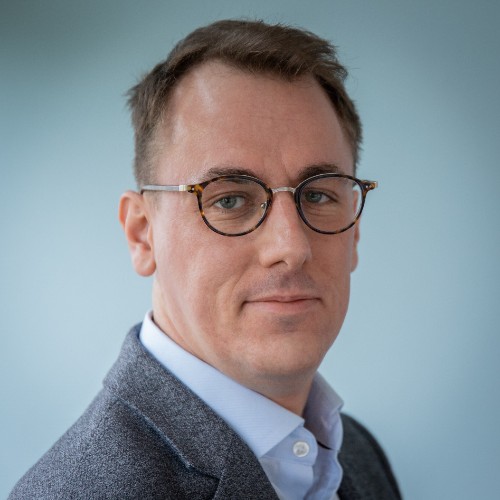
Chargé de recherche Inserm/Senior Research Associate
RDC Bâtiment Recherche
CHU de Nancy Brabois
54511 Vandoeuvre les Nancy
France
Biographie
The focus of my research is the use of modeling and signal-processing methods to extract novel clinical parameters from large databases of physiological signals, and the use of machine-learning techniques to provide predictive actionable information to clinicians. I have been applying these techniques to electrocardiographic (ECG) (and other physiological) data for more than 10 years.
My research project consists in developing novel machine-learning techniques combined with and/or inspired by modelling in order to create interpretable automatic decision-making systems in healthcare, with a particular focus to cardiovascular health data. Clinical assessment of the cardiovascular heath requires the acquisition of multimodal data, two of the most important modalities being Magnetic Resonance Imaging (MRI) and ECG. I therefore aim at developing tools for the joint analysis of electrophysiological and imaging data. To reach this goal, it is important to develop an efficient representation for imaging data, and electrophysiological data. These representations will then be used as a starting point for unravelling a joint representation of this multimodal data, therefore representing the physiological phenomenon in a personalised manner. These techniques and representations of data will offer solutions for concrete clinical problems such as better risk stratification for patients, whether for Atrial Fibrillation catheter ablation outcome prediction, and the risk of presenting Ventricular Tachycardia (VT).
This research project aims at finding new ways to fuse the information provided by the MRI and the cardiac physiological information provided by signals such as ECG (or Electrograms –EG-).
My research project is divided in three complementary axes focusing on: (i) analysis of electrophysiological data, (ii) MR image analysis and reconstruction accounting for motion, and (iii) investigating cutting edge machine learning approaches for bimodal representation of electrophysiological and imaging data.
Projets principaux :
1) Analysis of electrophysiological data:
Deep learning has shown great potential for the automated analysis of ECG signal as demonstrated by cardiologist-level performance for arrhythmia detection [1]. In my group, we also developed Convolutional Neural Network (CNN)-based approaches [2]. These methods apply a deep convolutional classification network with global output to a fixed-length window of a single ECG and are able to learn a multi-scale latent representation of the electrophysiological signal. We worked on new models that leverage forms of recurrent neural networks (RNN), allowing us to incorporate temporal information and therefore improve the detection of abnormal rhythm [3] (fig. 1). Nevertheless, such abnormal rhythm can be paroxysmal, meaning that short episodes of abnormality can be found in longer recordings. We will be adapting and developing new so-called attention models [4] for CNN-based ECG arrhythmia analysis, our method will enable the automatic detection and accurate localisation of arrhythmic events. A collaboration has already been initiated on these topics, with a German partner at the University of Luebeck with a joint direction of a Master thesis.
One of the major drawbacks of these approaches lies in the absence of interpretability; we therefore
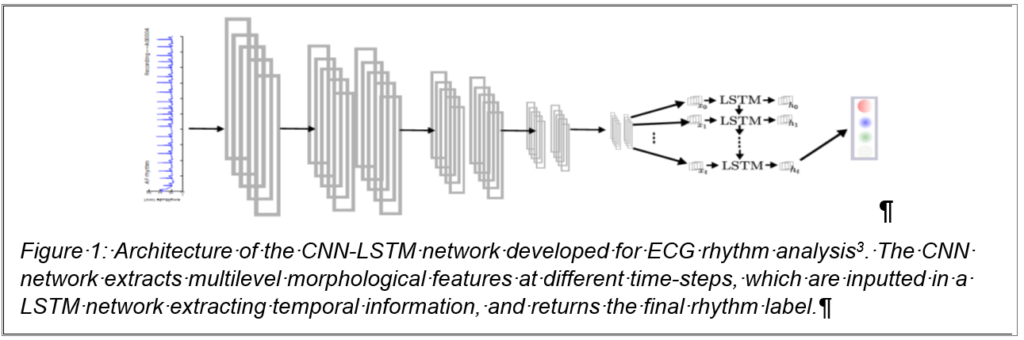
focused on developing new tools in order to better understand how the network was making its decision. The cardiologist was then provided with a rhythm and the regions of the data that was paramount to the decision-making. We will be furthering our quest for such interpretation tools during this project, one solution being the integration of physiological knowledge in the design of the network.
2) MRI image analysis and reconstruction integrating the motion
End-to-end deep learning approaches using CNNs have recently been proposed for medical image segmentation [5] (cardiac MRI), but require large amounts of training data and are affected by subtle changes in acquisition protocols. To obtain accurate segmentations generalizable to new patients that are robust against potential motion artefacts and consistent across subjects with respect to the underlying heart anatomy for subsequent motion tracking, current feed-forward CNN networks may be insufficient. We believe that accounting for the motion of the heart will help overcoming current limitations of end-to-end deep learning approaches. Recent work in cine-MR modelling demonstrated promising early results for clustering cardiac motion for dense fields [6], these dense fields could be used for simulation of pathological images of the heart (fig. 2) and such approach has been shown to be a very promising data augmentation procedure [7].
Nevertheless, the use of motion models could also be employed in order to automatically estimate
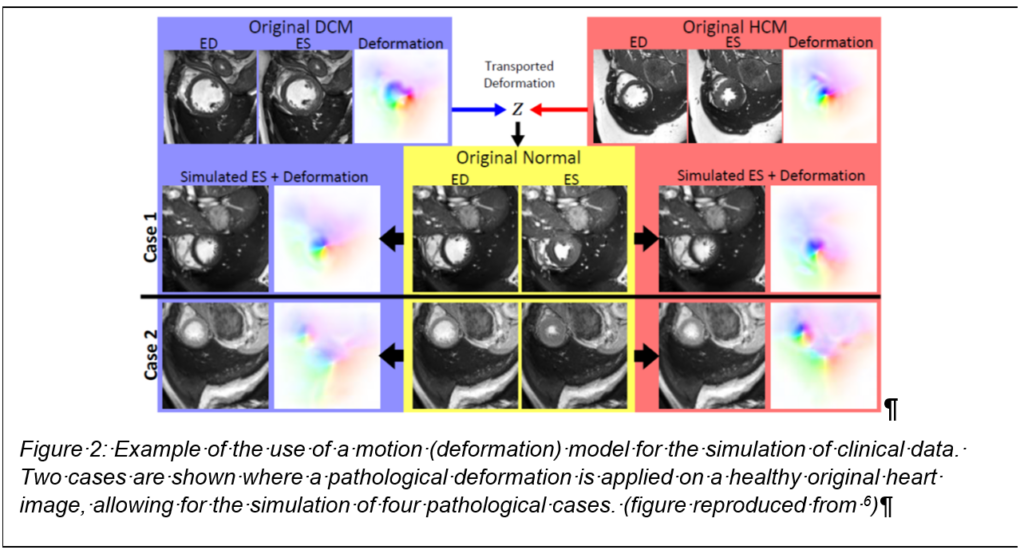
some physiological parameters, such as T1 map or perfusion parameters. Current approaches require the use of registration tools, which could be suppressed by incorporating the motion modelling in an automated end-to-end solution.
We will finally be exploring the possibility of incorporating these deformable models in an end-to-end deep learning reconstruction pipeline [8] in order to develop a new strategy for a 3D free-breathing acquisition of the heart. We will in this task investigate the use of reinforcement learning for optimal acquisition strategy [9]. Such studies might pave the way towards a fully autonomous acquisition of cardiac MRI data, and provide the radiologists with robust and accurate physiological parameter estimation for better diagnoses.
3) Multimodal deep learning
The final research axis will consist in the smart fusion of the information provided by MRI images and the electrophysiological signals. This project will first aim at solving specific clinical problems, and will serve as a proof of concept for a possible fully data-driven fusion of electrophysiological and images. Current approaches rely on the modelling of the propagation of the electrical activity, either modelling the electrical sources as dipoles in the brain or the heart (using the equivalent dipole layer source model) or modelling biopotential on the surface of the endocardium [10]. Such approaches have proved to be useful for some specific applications (planning of cardiac resynchronisation therapy), but do not seem to be accurately depicting more complex pathologies [11].
This research axis will rely on the developments of previous axes, and the architecture proposed for fusing images and electrophysiological will be inspired from previously proposed models.
This data-driven solution will aim at solving concrete clinical problems, such as the characterisation of VT isthmus. There is indeed an urgent need to develop a better risk stratification for patients at risk of VT [12], or for the prediction of AF catheter ablation outcome.
This joint representation of MRI (including motion) and ECG data will inherently include mechanistic and electrical properties of a patient’s heart. This approach constitutes a data-driven estimation of a digital twin of the heart, and provides insightful predictive and actionable information.
References:
1. Hannun et al., Cardiologist-level arrhythmia detection and classification in ambulatory electrocardiograms using a deep neural network. Nature medicine, 25(1), 65, 2019.
2. Clifford et al. « AF classification from a short single lead ECG recording: The Physionet Computing in Cardiology Challenge 2017. » Computing in Cardiology, 44 (2017).
3. Vogt Nora, MSc Thesis, CNNs, LSTMs, and attention networks for pathology detection in medical data, University of Lubeck, 2018.
4. Zhao et al.: « Diversified Visual Attention Networks for Fine-Grained Object Classification » IEEE Transactions on Multimedia, 19 (6) 1245 – 1256, 2017.
5. Oktay et al.: Anatomically constrained neural networks (ACNN): Application to cardiac image enhancement and segmentation. IEEE Transactions on Medical Imaging in print 2018.
6. Krebs et al., Unsupervised probabilistic deformation modeling for robust diffeomorphic registration. In Deep Learning in Medical Image Analysis and Multimodal Learning for Clinical Decision Support (pp. 101-109). Springer, 2018.
7. Corral Acero et al., SMOD – Data augmentation based on Statistical Models of Deformation to enhance segmentation in 2D cine cardiac MRI, FIMH 2019.
8. Zhu et al. « Image reconstruction by domain-transform manifold learning. » Nature 555.7697 (2018): 487.
9. Zheng et al., Reinforcement Learning for Online Undersampling Pattern Optimization, ISMRM, 2019.
10. Cluitmans et al. « Noninvasive reconstruction of cardiac electrical activity: update on current methods, applications and challenges. » Netherlands Heart Journal 23.6: 301-311, 2015.
11. Duchateau et al. « Performance and limitations of noninvasive cardiac activation mapping. » Heart rhythm 16.3 : 435-442, 2019.
12. Goldberger et al. « Risk stratification for sudden cardiac death a plan for the future. » Circulation 516-26, 2014.
Récompenses et nominations :
2020 top 10%, of high-scoring reviewers for NeurIPS 2020.
2017 1st Place, MICCAI Segmentation Challenge ‘Multimodal Whole Heart’ (only applied to MRI Images).
2016 Highlights of 2016, « Evaluation of the foetal QT interval using non-invasive foetal ECG technology » selected by Physiological Measurement.
2016 Highlights of 2016, « A practical guide to non-invasive foetal ECG extraction and analysis » selected by Physiological Measurement.
2014 Winner of Phase 3, of the Physionet/Computing in Cardiology Challenge: Robust Detection of Heart Beats in Multimodal Data.
2014 Bill and Gary Sanders poster award, at Computing in Cardiology.
2013 Unofficial winner of events 1 and 2, of the Physionet/Computing in Cardiology Challenge: Noninvasive Fetal ECG.
2013 Best poster award, at the SCMR/ISMRM Jointly Sponsored Workshop: New Horizons in High Field Cardiovascular MR: Promises and Progress.
2012 J.A. Lodge Award, from the Institute of Engineering and Technology (IET), for recognition of promising early career in Biomedical engineering.
2012 2nd prize for the best poster award, at the Workshop of Biomedical Signal Interpretation.
2010 Newton International Fellowship, University of Oxford.
Autres implications :
• Member of Computing in Cardiology
Publications
1. J. Behar, J. Oster, Q. Li and G.D. Clifford, « ECG signal quality during arrhythmia and its application to False Alarm reduction, » IEEE Transactions on Biomedical Engineering, 60, 1660-1666, 2013
2. J. Oster, J. Behar, O. Sayadi, S. Nemati, A.E.W. Johnson, G.D. Clifford, “Semi-supervised ECG Ventricular Beat Classification with Novelty Detection Based on Switching Kalman Filters, » IEEE Transactions on Biomedical Engineering, 62(9), 2125-2134 ,2015
3. J. Oster, O. Pietquin, M. Kraemer and J. Felblinger, « Nonlinear Bayesian Filtering for Denoising of Electrocardiograms acquired in a Magnetic Resonance Environment, » IEEE Transactions on Biomedical Engineering, 57, 1628-38, 2010.